Artificial Intelligence (AI) is everywhere these days. It’s helping us order our groceries, write emails, and even recommend the next binge-worthy TV show.
But one area where AI is making waves—and perhaps raising a few eyebrows—is recruitment. AI recruiting tools now promise to sift through resumes, predict candidate success, and save companies loads of time in the hiring process. What could go wrong, right?
Well, quite a bit, actually.
These shiny new tools aren’t infallible. While they might seem like the perfect solution to streamline hiring, they can sometimes perpetuate bias—resulting in what experts call AI recruitment bias.
This issue isn't just a tech problem. It affects businesses, job seekers, and anyone who believes hiring should be fair. And as we dive into this topic, you'll see how bias sneaks in, why it matters, and what we can do to stop it.
Table of Contents
‍
The rise of AI in recruitment: Boon or bane?
Let’s start with the basics. AI in recruitment has a lot of upside. Imagine a tireless assistant who can analyze hundreds, even thousands, of resumes in the time it takes to grab a coffee.
No more hours spent reading through cover letters or agonizing over which candidate best matches the job description. With AI, the process becomes faster, more efficient, and likely much smarter.
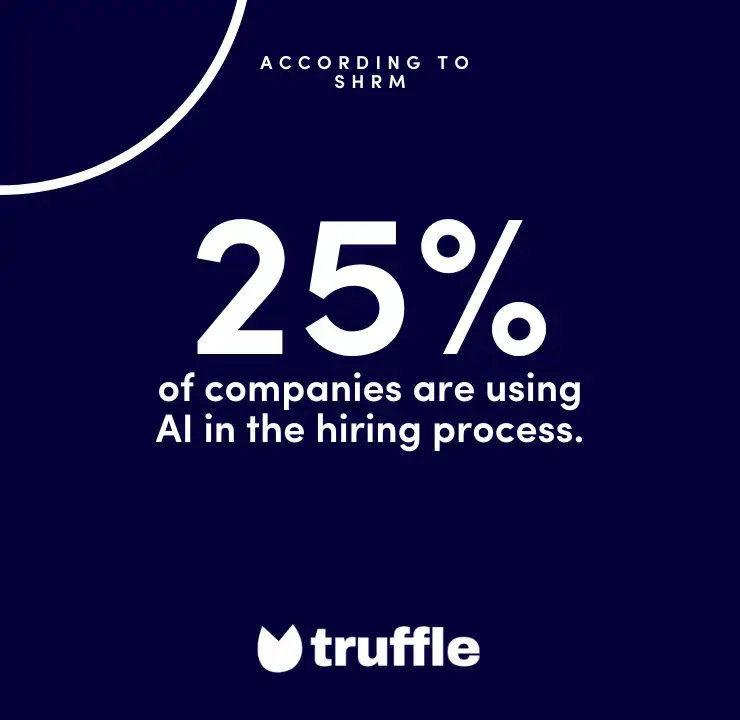
Just like any powerful tool, AI comes with its quirks. And here’s the catch: AI systems learn from the data they’re fed. If the data has biases—let’s say it leans toward favoring a particular gender or age group—guess what? The AI tool will learn to favor those same biases.
It’s the tech equivalent of monkey see, monkey do. And this can lead to some eyebrow-raising results. Take a well-known example where a tech giant (yes, that one) built an AI to screen resumes.
The system was trained on resumes from the past decade, which, naturally, were dominated by men. The result? The AI ended up favoring male candidates. In some cases, it even penalized resumes that contained the word "women."
So while AI can be a huge boon for recruiters, it can also be a bane if not handled with care. It’s a delicate balance—you need to know when to hit the gas and when to tap the brakes.
What exactly is AI recruitment bias?
You might be wondering, how does this happen? How can AI—a machine built to make decisions based on data—end up biased?
Well, it all comes down to the training data. Think of AI as a student. It learns from the information it’s given, and if it’s fed biased data, it will adopt those biases like a sponge. This is what we call AI recruitment bias. It happens when these tools make decisions that reflect the skewed patterns of the past.
Let’s say an AI tool is trained using data from a company that historically favored male candidates. Even if gender is irrelevant to the job, the AI might still lean toward male candidates simply because it has learned that men were previously hired more often.
This isn’t because the AI is consciously discriminatory—AI, after all, doesn’t have a conscience. But it can only work with the information it’s given. As the old saying goes: garbage in, garbage out.
This bias can snowball, too. It doesn’t just limit individual candidates. It can affect entire companies by reinforcing a lack of diversity and perpetuating the same homogenous work environments that have been problematic for decades.
When AI Recruitment goes wrong: Real-world stories
Let’s face it—recruitment bias isn’t some abstract, theoretical issue. It’s real, and it’s already happened.
Remember that tech company we mentioned? They’re not the only ones who’ve stumbled with AI recruitment. Another company used an AI tool to screen applicants and thought, “What could go wrong?” Plenty, it turns out. The system had been trained on data from their top performers, which were overwhelmingly young. As a result, the AI began favoring younger candidates and inadvertently practiced age discrimination.
In other cases, AI tools have given higher scores to candidates from certain schools or backgrounds while sidelining those with more diverse experiences. Why? Because the data suggested these "elite" candidates performed better in the past.
But here’s the thing—correlation doesn’t always equal causation. Just because someone attended a prestigious school doesn’t mean they’re inherently better suited for a job. But try telling that to an AI that only knows how to pick up patterns.
These examples serve as a wake-up call: AI might be smart, but it isn’t perfect. And when it makes mistakes, the results can be expensive.
The data dilemma: Garbage in, garbage out
Here’s where things get a little tricky. AI recruitment tools are essentially pattern-recognition machines. They look at what worked in the past and try to replicate that success. But if the past was biased, the future will be too.
The phrase “garbage in, garbage out” comes into play here. If the data you feed your AI system is skewed—say, if it comes from an industry that’s been historically dominated by one gender or race—the system will likely reproduce those same biases. It’s not rocket science. It’s just how machines work.
So, how do we avoid the garbage trap? By using diverse, representative data. AI systems need to be fed information that reflects the broad spectrum of humanity—not just the small, historically privileged sliver that dominated certain industries.
This means incorporating data from different genders, races, backgrounds, and experiences. And no, it’s not just about being politically correct—it’s about building a tool that genuinely reflects the world we live in today.
But data alone isn’t enough. You also need people—human beings with empathy, awareness, and the ability to see beyond algorithms—at the helm, overseeing the process and making sure the AI doesn’t go rogue.
Navigating the ethical and legal questions
And then, there’s the ethical and legal side of things, but these aren’t insurmountable challenges. AI recruitment, when used thoughtfully, actually offers an opportunity to improve fairness and equality.
Unlike humans, AI doesn’t get tired or make decisions based on gut feelings or unconscious bias. It evaluates candidates based on data, which, when managed correctly, can lead to more objective hiring decisions.
For companies using AI to sort through applications, it’s crucial to ensure the system is designed to minimize bias. With the right checks in place, AI can actually help reduce discriminatory practices by standardizing how candidates are assessed.
In fact, many see AI as a tool that can help ensure compliance with Equal Employment Opportunity laws by providing consistent, data-driven evaluations. If anything, AI can help prevent the very biases that human-driven processes might overlook.
Ethically, using AI doesn’t replace human oversight—it enhances it. AI can handle the heavy lifting, analyzing huge datasets that would be overwhelming for humans to manage. But ultimately, human judgment remains an integral part of the process, ensuring that fairness is upheld. Rather than posing an ethical dilemma, AI offers an opportunity to rethink recruitment in a way that is both more efficient and more equitable.
These are exciting challenges to tackle as AI takes on a bigger role in recruitment—and in our daily lives. With thoughtful implementation and a focus on continuous improvement, AI can actually help us build a more fair, transparent, and inclusive hiring process.
Strategies to combat AI recruitment bias
Now that we’ve laid out the problem, let’s talk solutions. Because, spoiler alert, AI recruitment bias isn’t an unsolvable issue. There are ways to combat it—and to use AI for good, without letting it reinforce old prejudices.
Regular audits and accountability
First things first: regular audits. Just like you’d audit a company’s finances, AI tools need to be checked, rechecked, and checked again. You need to make sure the system isn’t drifting into biased territory. This kind of accountability ensures that the algorithms stay on track and continue to promote fairness.
Diversifying development teams
It’s not enough to have diverse data. You need diverse people building the AI systems in the first place. A team of developers with different backgrounds and perspectives is less likely to overlook biases because they bring a broader range of experiences to the table. The more perspectives, the better the outcome.
Enhancing transparency and communication
One of the best ways to build trust in AI recruitment is through transparency. Let candidates know how AI is being used in the hiring process. Be upfront about what’s being measured, why it matters, and how decisions are made. Clear communication can go a long way in building trust and preventing the AI from becoming a "black box" that nobody understands.
Emphasizing human oversight
And finally, human oversight is key. AI should never be a set-it-and-forget-it tool. You need real people monitoring the process, stepping in when the AI makes mistakes, and ensuring that fairness prevails. AI may be powerful, but it’s not infallible. And sometimes, it takes a human touch to get things right.
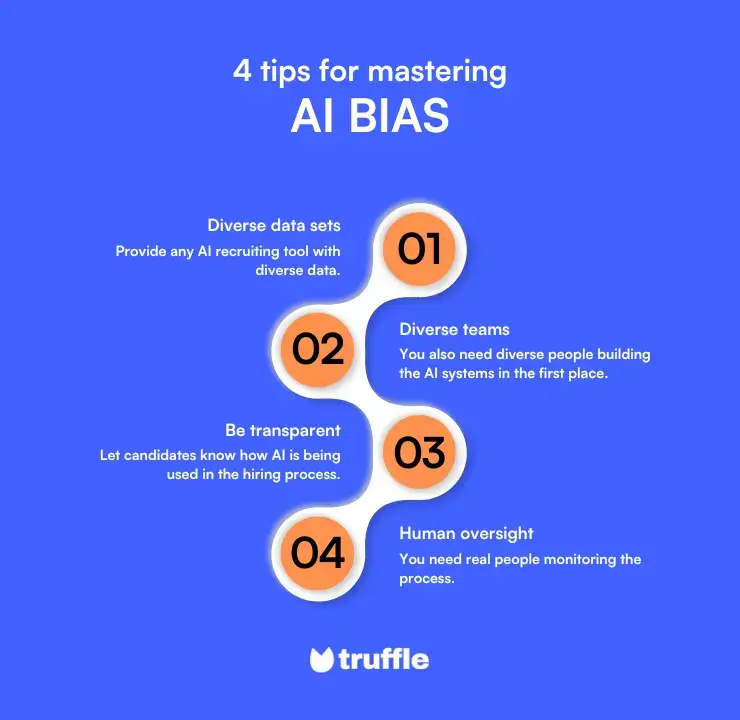
The role of continuous learning in AI recruitment
AI isn’t a one-and-done solution. It’s constantly evolving, and that means it needs continuous learning. As society changes, so do the patterns of what makes a good hire. And your AI needs to keep up with these changes. Think of it as updating your wardrobe: what worked five years ago might not cut it today.
AI systems should be regularly retrained, using fresh, diverse data that reflects the evolving job market. This ensures that the tools remain relevant, effective, and—most importantly—fair. After all, fairness in recruitment isn’t a static goal. It’s something that requires constant attention and adjustment.
‍